Practical considerations
The energy sector has historically been behind the curve compared to other sectors such as telecoms and finance when it comes to the integration of digital technologies. The use of AI in the energy sector is no exception, however this is now rapidly developing. A key challenge for the industry is the need to navigate the complex and rapidly evolving energy sector and its intricate regulatory landscape, which is undergoing a generational shift, as the UK looks to develop an energy market increasingly based on low carbon generation.
Part of the challenge stems from the fact that the UK is transitioning from a historic system based on centralised and predictable generation, with largely unidirectional flows of energy from the generator to end users. The new energy landscape has increasing reliance on disaggregated and intermittent generation and increasing complexity of both generation and demand profiles. This results in a step change in the amount of data and data points measuring generation and supply of electricity, and a need to balance increasingly complex supply and demand profiles across all scales. Digitalisation and the use of artificial intelligence has emerged as a potential solution in the energy sector to address this challenge, allowing vast amounts of data to be processed and processes to be automated and respond to market signals in real time.
The energy sector is investing heavily in AI for a wide range of uses, including:
- Forecasting and optimisation: AI enhances energy supply and demand forecasting and optimises thermal and renewable power generation as well as Energy Storage.
- Smart technology: utilises machine learning for energy efficiency monitoring (smart meters) and grid management (smart grids);
- Oil and gas: improves drilling accuracy and operations using data analytics;
- Diagnostic and predictive maintenance: uses AI for data analysis, fault prediction, and maintenance scheduling;
- Weather prediction: assists with renewable energy production;
- Market efficiency: enhances efficiency in energy markets and grid management.
Key challenges of AI in the Energy sector
When it comes to AI in the energy sector, energy businesses must consider certain challenges for successful integration. A few common challenges to be aware of include:
- Ensuring data accuracy and reliability when AI models are trained on vast data sets. Issues with data access and standardisation has meant that there are limits to what can be achieved, but the industry is moving toward more open-source data sharing standards to combat this and unlock the potential of energy data to provide both individual services and whole-system efficiencies.
- Data security and protection – large volumes of energy system data will relate directly to household consumption and ensuring robust process are in place to put appropriate controls on access to and use of data will continue to be a key consideration for the foreseeable future.
- IP considerations – data protection under various IP laws, copyright as well as database rights.
- Staying up to date with the ever-changing technology uses and trends in an industry that is based on assets with long lifecycles and multi-year/decade investment cycles.
- Contract documents in the renewable energy sector may be bespoke and unique to individual projects or stakeholders. This means there are significant parts of the industry without long-term market standard positions, or where contract terms are kept highly confidential. This makes AI driven document production more challenging due to insufficient training data being freely available.
- Regulatory frameworks vs technology: Given the speed with which new technology is introduced, it is unsurprising that rules and regulations lag behind. This is a real concern in highly regulated energy markets and carefully balanced energy systems (electricity and gas grids in particular). There is a risk that regulatory frameworks are insufficiently flexible or otherwise unfit for purpose to allow the full benefits of new technologies to be realised. Of at least equal concern is the risk that they are unable to prevent abuse or mitigate market shocks (such as price spikes) when technology allows decisions to be taken quicker than rules and regulators can respond.
Legal considerations
Risks
- Cybersecurity and data protection issues – the World Energy Council has warned that increasing interconnection and digitisation of the industry makes it a prime target for cyber criminals, state-sanctioned cyber-attacks, terrorists and hacktivists. Such attacks can disrupt energy supply, lead to data breaches, and compromise sensitive information.
- Miscorrelations due to insufficient training, data or coding mistakes, for example, faulty AI predictions in demand forecasting could lead to either a surplus or shortage of energy, affecting grid stability.
- Liability where things go wrong – determining liability in the event of AI system failures or incorrect decisions can be complex. Clear legal frameworks are required in order to address who is accountable when AI systems cause harm or loss.
- Questions about accountability for public spending, energy prices or outages where AI is used.
- AI itself uses up a lot of energy and this will increase as AI grows. Corporations will need to make informed decisions when choosing AI providers, partnering with those who energy-efficient and sustainable.
Key takeaways
The integration of AI into the energy sector clearly presents significant opportunities and enhancements, however, these advancements come with practical and legal challenges that must be carefully managed. Ensuring robust cybersecurity measures, addressing data privacy concerns, and maintaining compliance with regulatory frameworks within the sector is critical for mitigating the risks that have been discussed above.
As the energy sector continues to evolve, propelled further by AI, stakeholders must balance innovation with diligent oversight. By adopting best practices and ensuring collaboration between the technologists, regulators and industry leaders, the energy sector can harness the full potential of AI, whilst safeguarding against its inherent risks. This approach will not only enhance the reliability and efficiency of energy systems, but also contribute to a more sustainable and resilient future for energy.
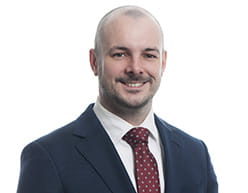
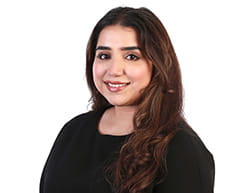