The adoption of AI and Generative AI across all businesses has moved from a question of "if" to "when". The growing incentives to adopt and explore the wide-ranging capabilities of both will naturally come with risks for a wide range of stakeholders across businesses.
The purpose of this T&H AI series over the coming weeks is to provide an overview of the legal implications posed by AI and Generative AI, the practical considerations of using this technology, and how we can offer our expertise through innovative applications.
The basics
What is AI?
What does 'Artificial Intelligence' actually mean? To its core, AI is a machine-based system which enables computers and machines to simulate human intelligence through various elements of autonomy. The comparison to human intelligence derives from the fact that AI algorithms replicate the decision-making processes of the human brain, in the sense that it can learn from accessible data, produce outputs such as classifications, predictions, decisions and content which will become more increasingly accurate over time.
The term 'machine learning' is the process of improving the AI systems' performance with experience and by training it with 'input data'. It is considered a subset of AI. The AI system will proceed to learn and improve on its own with neural networks, a series of algorithms mimicking the human brain. Machine learning works well with data that is constantly evolving or where the nature of tasks required from the AI system are susceptible to change.
The rise of generative AI
Generative AI is a groundbreaking subdivision of Artificial Intelligence. Definitions of what this actually is vary, but the EU AI Act has defined Generative AI as a type of foundation model used in AI Systems "specifically intended to generate, with varying levels of autonomy, content such as complex text, images, audio, or video".
This leads us to the question, what are foundation models? Foundation models provide wider AI functionality through a series of neural networks with the ability to analyse unstructured data and learning to generate specific outputs. These models can be categorised by: Single Modals, which can receive a single source or type of data and generate content using text; and Multi-Modals which can receive multiple sources and types of data, including video, images, audio and text that generate detailed perceptions of the data which have been input.
The major global shift and focus on AI can be attributed to the rise of Large Language Models (LLM) such as OpenAI's ChatGPT. LLMs are a type of foundation model and Generative AI which transformed the potential of AI for two key reasons:
- Language Complexity: LLMs can learn language, apply the context and generate creative outputs; and
- Pre-trained on large quantities of data: LLMs can utilise the analysis on vast quantities of varied data and the models can be employed for a wide range of tasks.
Language underpins every aspect of how a business operates on a day-to-day basis, whether that is through emails, contracts, document management systems, videos or audio.
Generative AI is transforming businesses across sectors. In healthcare, Generative AI is revolutionising the patient-clinician experience with tools that can transcribe patient consultations and generate preliminary clinician notes. AI innovation in the finance sector has included applications such as algorithmic trading, gathering market intelligence, monitoring financial performance and detecting data anomalies to prevent fraud. The adoption of Generative AI across all sectors will become inevitable and will ultimately transform the way business is conducted.
The application of AI in the workplace has the potential to speed up routine aspects of daily tasks across a wide range of businesses. The integrated use of AI allows for specialist tasks to be completed cost-effectively, for example summarising documents with specialist language at faster speeds. Accenture has predicted that 40% of all working hours can be impacted by LLMs. The collaboration between AI and human input will allow for employees to delegate certain tasks to focus their time on more important aspects of their work, enabling businesses to deliver time and cost-effective services.
The barriers to adoption of Gen AI
Accuracy: Perfect accuracy and reliability of any AI system's final output cannot be guaranteed and businesses must be cautious of this risk. The quality of the output will vary on specific factors such as: the type of data being used; how the data is being used; and the type of task required from the AI System. For instance, the generative AI's algorithm can present false content known as 'hallucinations' which can be highly damaging to a company relying on the output for decision making and not implementing any human interaction. Ultimately, AI should be treated as a collaborative tool with caution, employees should always check the final output and monitor the type of data the algorithms have been trained on.
Ethical Use: Ethical concerns have been raised globally as there is potential for AI Systems to be embedded with bias and discrimination, consequently threatening fair process and in some cases human rights. Bias can infiltrate the AI System during the input of data, training or output stages of its lifecycle. For instance, representation bias could be evident at the 'input stage' if an algorithm is only fed data which is either unrepresentative or overrepresented of certain social groups, resulting in social inequality. Furthermore, the data itself could contain bias which the algorithm learns and copies. From a recruitment perspective, if the AI is only trained with racially biased data, the bias will be evident in the decision it makes on a potential employee. This threat could exacerbate existing inequalities and prejudices across marginalised groups and lead to detrimental impacts on individuals.
These concerns led to UNESCO producing the first global standard on AI Ethics which was adopted by 193 Member States, including the UK, at UNESCO’s General Conference in November 2021. The recommendation highlights core values such as protecting human rights, dignity, diversity and inclusion of people to be found in the foundations for all AI. The UNESCO "Women4EthicalAI' expert collaborative platform is one of the results of this recommendation which aims to advance gender equality in the design and deployment of AI Systems. Fairness must be ensured by identifying and mitigating biases from the data used by AI Systems in order to produce reliable final outputs.
AI Security: AI is vulnerable to attacks in its security similar to traditional computer systems and cybersecurity. There are multiple ways AI can be attacked: the outputs can be manipulated to lead to harmful or inaccurate outputs and information can be stolen.
Adversarial attacks are designed to lead the AI model to make a mistake and cause harm. For instance, 'data poisoning' is where new data is maliciously injected into the dataset when it is being trained which enables attackers to manipulate the models future actions. An example would be introducing harmful images and classifying them as safe, so that the AI model will learn this and apply it to similar images. A further method of attacking is 'model extraction' which enables attackers to reverse engineer the model by feeding it inputs and tracking outputs to expose sensitive information. This can be dangerous for businesses if the AI model holds proprietary or classified information that cannot be shared publicly.
The law
There has been a mixed approach worldwide in relation to regulating the AI phenomenon, from legislative frameworks, voluntary guidelines, national policies and the creation of regulatory bodies. The rapidly evolving nature of AI has posed a regulatory challenge in many jurisdictions but there have been differences in national approaches.
The UK has taken a 'pro-innovation' approach to AI regulation driven by the Department of Science Innovation and Technology (DSIT). The AI Regulation White Paper published in 2023 and subsequent government response introduced a framework which applies a cross-sectorial, principle-based and non-statutory approach to AI. The cross-sectorial principles for existing regulators to integrate within their remits include: safety, security and robustness; appropriate transparency and explainability; fairness; accountability and governance; and contestability and redress.
Regulators, such as the Financial Conduct Authority, Information Commissioners Office and the Office of Communications responded by updating their strategic approaches to AI to align with this framework which were published by DSIT. On the other hand, Lord Holmes aims to re-submit the private member's bill 'Artificial Intelligence (Regulation) Bill under the new Labour government with the belief that legislation is imperative .
The EU has taken a comprehensive legislative approach in contrast the UK's position. The EU AI Act is the world's first legal framework for the regulation of AI through a 'risk-based' system. Whereas the US has taken a lighter approach by introducing mandatory reporting requirements for foundation models which pose a security risk to the country.
However, the UK's position will soon change. The prospect of AI legislation in the UK was made clear by the new Labour government in its election manifesto, as it proposed to "ensure the safe development and use of AI models by introducing binding regulation on the handful of companies developing the most powerful AI models". The potential 'AI Bill' was not announced as anticipated during the King's Speech on 17 July 2024. However, it was stated that the Government would "seek to establish the appropriate legislation" and in the interim, the Government has announced the introduction of a Cyber Security and Resilience Bill, to address the increasing risks of cyber-attacks, and a Digital Information and Smart Data Bill.
Looking ahead
This AI series will explore a range of specific legal and practical considerations businesses need to be aware of ahead of the inevitable adoption of AI and Generative AI into their strategies. The series will commence with a deep dive into AI and intellectual property.
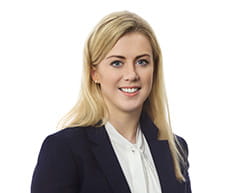