Integrating AI and generative AI into business strategies is no longer a choice for private equity firms, it is imperative to maintain a competitive edge.
There are a range of use cases across an investment lifecycle; from sourcing and conducting due diligence, to analysing data and financial performance, portfolio management and ultimately exit. However, harnessing this new technology comes with inherent risks which should be considered and managed carefully. This article explores some of the benefits and challenges which PE firms might face when integrating AI at different stages of an investment.
Investment
Investment sourcing and analysis for PE firms is traditionally the result of utilising well-established relationships, networks and extensive market research. AI and generative AI tools can enable a quicker, wider and more proactive approach to identifying and evaluating potential investment opportunities. By integrating large language models into a PE funds’ knowledge base, AI tools can analyse huge quantities of data from multiple sources. This can include financial data, company data and market data (such as analysis on market conditions, sector trends and industry reports). Once the data is collected, machine learning algorithms use predictive analysis to identify attractive investments, for example through consistent revenue growth or low debt levels. AI tools could also be used for scenario analysis, at Investment Committee stage, where AI algorithms predict impact on an investment’s potential performance, from looking at interest rates to economic growth.
The private equity landscape remains competitive with funds competing for the highest quality assets. The ultimate prize is always an off-market transaction, where a fund can avoid becoming involved in an auction process; consequently, funds which can identify investment opportunities early by integrating the benefits of AI tools, whilst mitigating the potential risks, are likely to find themselves with an edge. However, it remains true that successful transactions always have, and always will be, forged out of strong personal relationships between investors, sellers and management teams. Whilst AI tools might assist with target identification and scenario planning, there is no replacement for that human connection.
Risk management
Once an investment has been made, the focus shifts to managing portfolios to ensure growth and maximise potential returns. Generative AI has the potential to play an integral role in this process by offering advanced analytical tools and insights that support strategic management and improve decision-making. For instance, it can utilise historical data and machine learning models to predict the performance of portfolio companies. Some firms have already leveraged generative AI capabilities across their portfolios to identify, for example, those that might require further funding to support them through challenging upcoming periods. AI can predict declining sales performances based on market conditions and anticipate shifts in customer behaviour. By predicting performance, PE firms and management companies can take proactive measures to mitigate potential risks or re-evaluate the lifespan of investments.
Most private equity firms are in the early stages of integrating generative AI into their portfolio management process, and it's important to understand the challenges associated with greater adoption. One of the primary concerns is the accuracy of AI prediction, which is heavily dependent on the data used. If the input data fed into the AI to develop its algorithms is biased or outdated, it can lead to incorrect predictions, poor decision-making, and reputational damage. Furthermore, using historical data could also fail to account for new and unforeseen market trends and conditions, resulting in unreliable predictions that do not accurately reflect the market dynamics at the time of usage. There is also a risk of sharing sensitive information and breaching confidentially if AI models use input data collected from portfolio companies or historic investments to further train their algorithms. This is especially the case where the AI tool is not proprietary to the PE firm and may be used by other third parties. If proprietary information, confidential investor, or client data becomes available to other users of the AI tools, including competitors, this could lead to both financial and reputational damage.
Ultimately, using AI and generative AI during the holding period of an investment can enhance risk analysis and drive a forward-thinking approach to portfolio management; but it will be important for the investment team to challenge and interrogate the outputs from any AI tools being used and to remain aware of the importance of taking steps to ensure confidentiality of proprietary information.
Exit phase
The exit phase is an essential phase for realising returns on investments. Generative AI can assist with crafting a successful exit strategy by helping PE firms assess the optimal time to exit an investment. A data driven approach can provide valuable insights during negotiations and help firms to secure the best possible deal. By analysing potential buyers, current market conditions and investor sentiment, AI can streamline the exit process and ensure that firms are maximising the returns on their investments with greater efficiency. The time saved can be re-distributed into human creativity, in discovering new investment opportunities.
Using generative AI during the exit phase introduces its own set of challenges, such as ensuring the complete and seamless integration of this new technology with the traditional investment process and decision-making frameworks. It is essential that generative AI compliments rather than disrupts existing practices. Poor integration could lead to inefficiencies and slow down the exit process. We expect that most firms will embrace a phased implementation of generative AI; allowing teams to gradually adapt, trust and learn to use the technology effectively alongside current frameworks.
Practical considerations
To successfully utilise generative AI as a private equity firm, there are a few practical considerations which should be implemented to assist in mitigating the risks.
Data: Firms should ensure input data is current, comprehensive, and free of any bias. Ensuring models are being updated regularly is essential to account for sudden changes in the market and to prevent a one size fits all approach. In addition, teams should be aware of the limitations of AI and its potential lack of accuracy. Firms should therefore use generative AI as one of many inputs in the lifecycle of a portfolio rather than relying solely on the technology and use the technology to supplement human input, not to replace it.
Policies and procedures: Establishing AI risk management systems will ensure that PE firms can adopt AI tools safely. For instance, introducing strict internal governance policies and conducting regular security audits are essential to protect sensitive data and ensure confidentiality, and ensuring that those policies and procedures are adopted across the business.
Summary
AI has the potential to transform the private equity industry by streamlining deal sourcing, enhancing portfolio management and supporting exit strategies. The integration of AI can provide an advantage amongst competitors, and firms must embrace the disruption that AI brings and naturally evolve alongside AI, as its capabilities continue to grow.
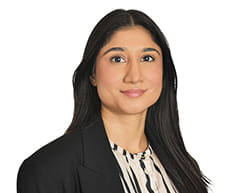